Ms. Zoja Scekic, a young researcher on HPC4S3ME project, defended her MSc thesis “Deep learning and applications in energy sector” today. This is one of the main project outputs in capacity building aimed at HPC/AI skills for applications in priority domains of Montenegrin S3.
ABSTRACT – This master’s thesis explores the application of advanced deep learning models for predicting day-ahead electricity prices, focusing on the accuracy and efficiency of these models compared to traditional forecasting methods. With the increasing integration of renewable energy sources and the growing complexity of electricity markets, accurate price forecasting has become crucial for market participants, grid operators, and policymakers. The research is structured around four case studies, each employing different deep learning techniques, such as Artificial Neural Networks (ANN), Long Short-Term Memory (LSTM), Convolutional Neural Networks (CNN), and hybrid models like CNN-LSTM. Despite the promising results, the research recognizes limitations related to data quality, model complexity, and computational resource requirements. The study emphasizes the need for further research into optimizing model efficiency, integrating more diverse data sources, and expanding the applicability of these models to different energy markets.
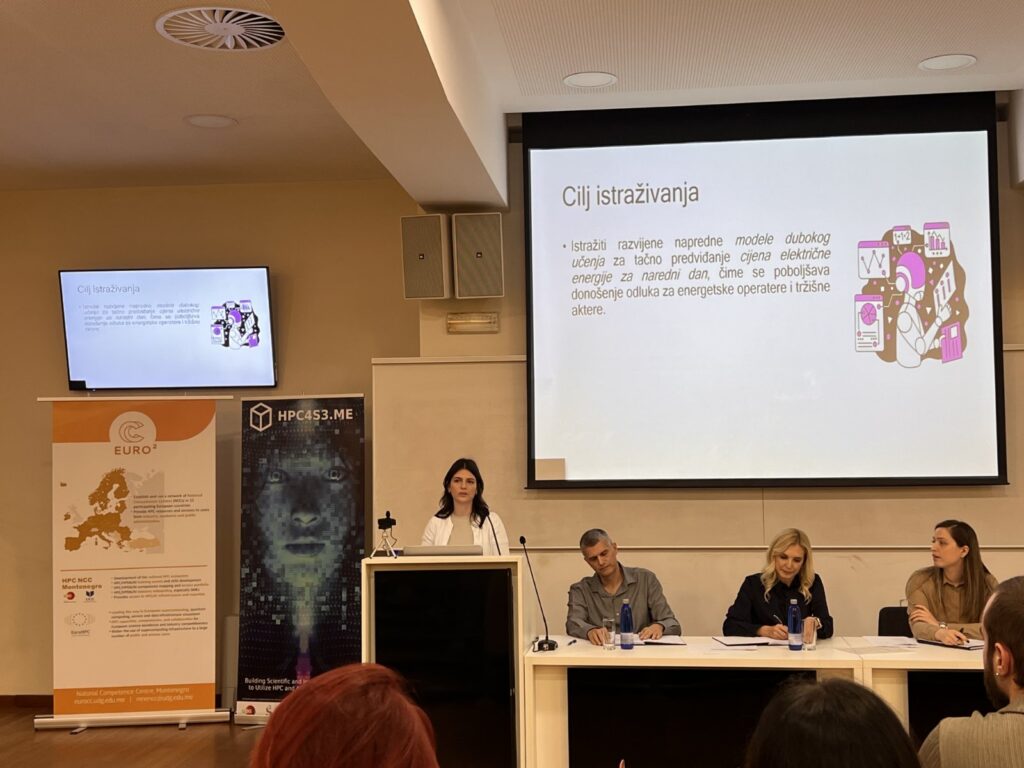
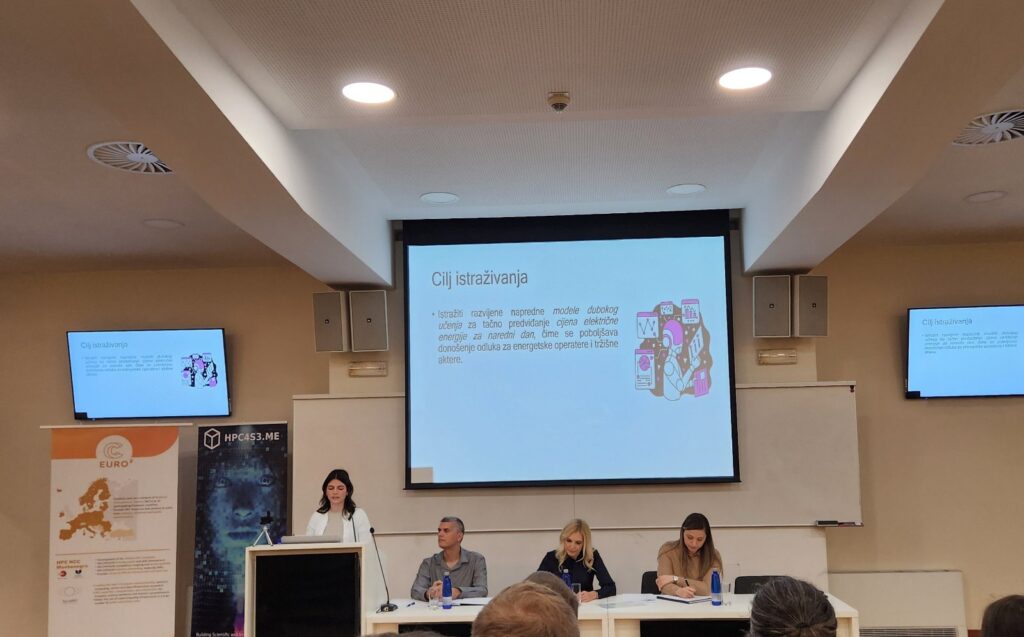
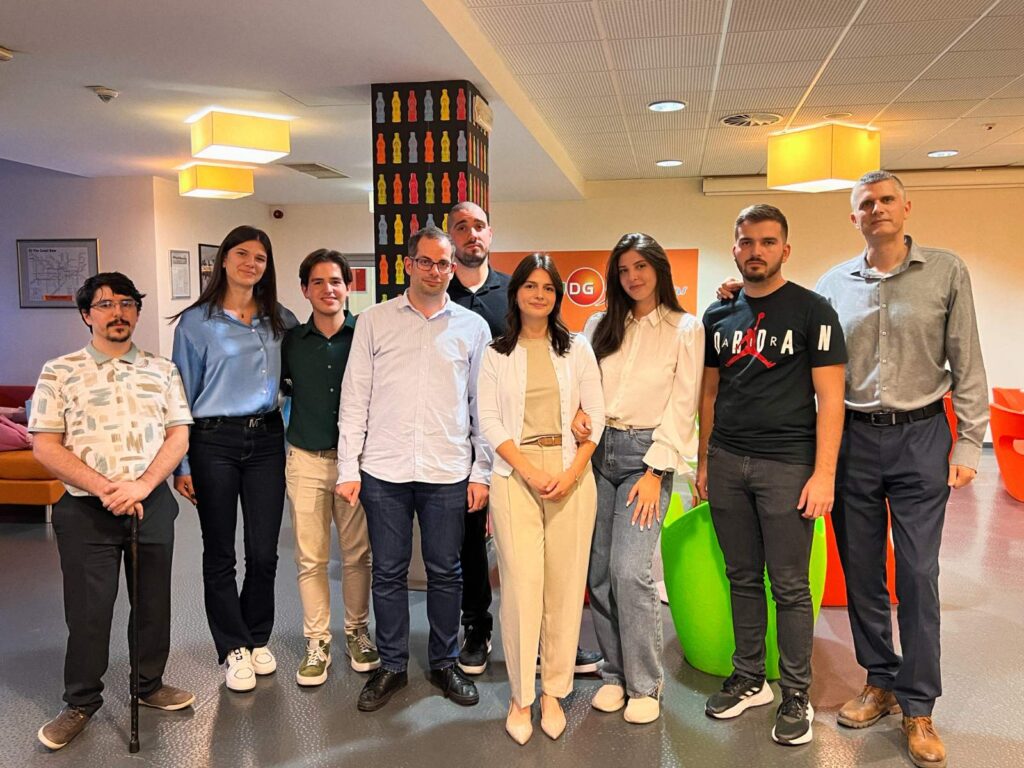