Mr Dejan Babic presented the research paper “Ten Year Cardiovascular Risk Estimation: A Machine Learning Approach” on a joint event CMBEBIH & MEDICON 2023 which held place in Sarajevo, Bosnia and Herzegovina from September 14th to September 16th. The paper presented a comprehensive analysis of the use of Machine Learning and Artificial Intelligence techniques in the sense of predicting the risk, and therefore evaluate their potential in identifying patients with possibilities of developing cardiovascular disease.
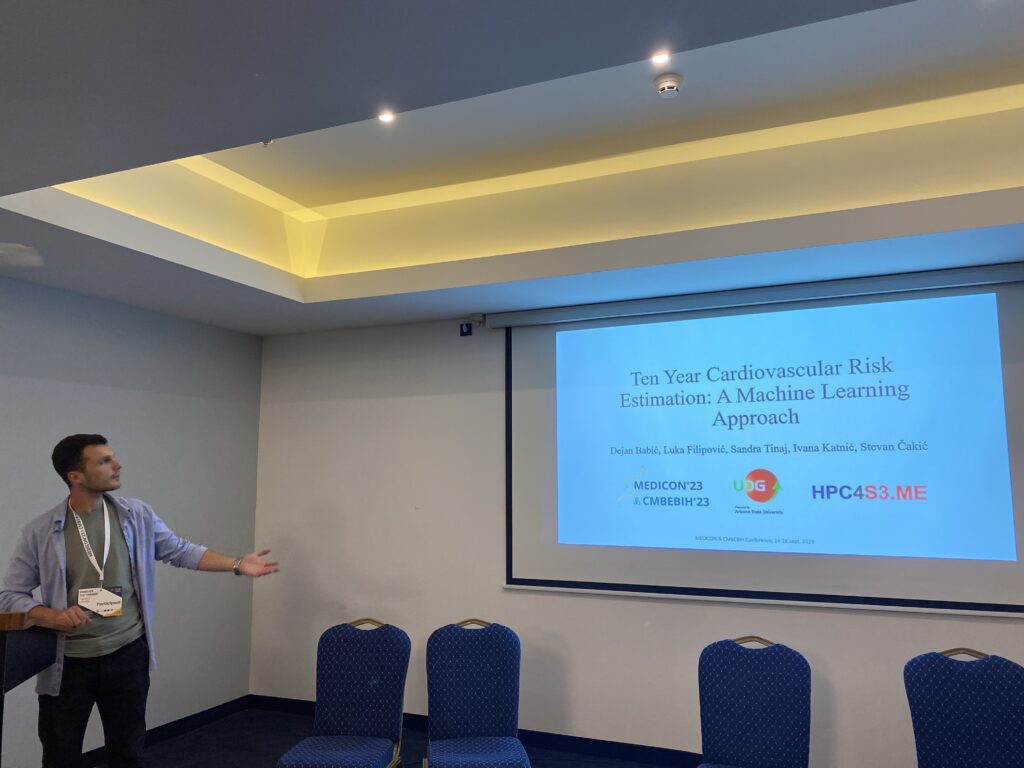
ABSTRACT – Cardiovascular disease is one of the most common causes of death in the world. Usual practice in medicine is estimation of 10 years risk of patients developing cardiovascular disease. This practice enables taking preventive measures such as early medical treatments and lifestyle changes. The key step is to accurately assess the risk where the patients can be treated accordingly. With advancement of Artificial Intelligence and ML in medicine, this study aims to present a comprehensive analysis of the use of these techniques in the sense of predicting the risk, and therefore evaluate their potential in identifying patients with possibilities of developing cardiovascular disease. First, an analysis of the working dataset is provided. Then, various traditional classification models are implemented and trained on the dataset, and their performance is compared. The classifying models used in this research include SVC, KNN etc. The results of this study are presented in systematic comparison, where the performances of all the models are represented with discussion about challenges and possible limitations of the data regarding this particular problem. Normalization and oversampling techniques were used and reduced the overfitting problem.
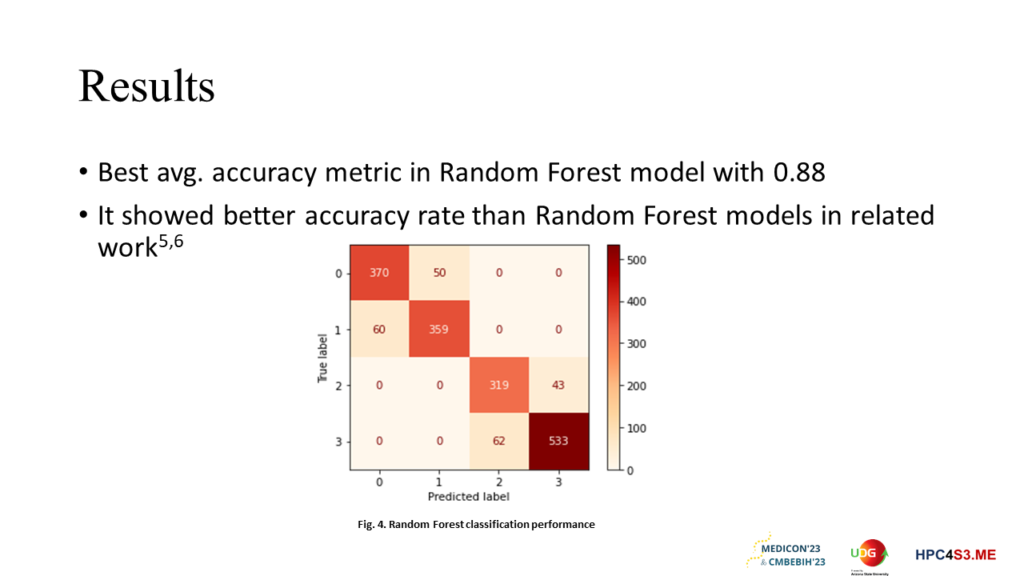